GPT Reviewer: An Analytical Perspective
OpenAI has unveiled CriticGPT, a groundbreaking model designed to pinpoint flaws within GPT-4’s underlying code. This innovation is part of an initiative to incorporate these models into the RLHF alignment process, enhancing the supervision provided by humans for complex AI-driven tasks.
Rooted in the capabilities of GPT-4, CriticGPT offers critical assessments of ChatGPT’s outputs, thus aiding human trainers in recognizing errors during the RLHF phase.
Introducing CriticGPT
Emerging from the framework of GPT-4, CriticGPT is engineered to detect errors in ChatGPT’s generated code. Studies have shown that individuals utilizing CriticGPT for code review boast a 60% improvement in performance versus those without such support. The ongoing development aims to weave CriticGPT and similar models into the fabric of the RLHF labeling workflow, offering direct AI assistance to trainers. This augmentation is set to refine the assessment of outputs stemming from sophisticated AI mechanisms, a task that poses a challenge without the availability of advanced tools.
The GPT-4 family of models, which includes ChatGPT, is characterized by its useful and engaging design, leveraging “Reinforcement Learning from Human Feedback” (RLHF). A pivotal element of this methodology is gathering AI trainer comparisons, which involves evaluating various ChatGPI responses against each other.
As technological advancements drive improvements in reasoning and model behavior, ChatGPT’s accuracy escalates, with mistakes becoming increasingly nuanced. Such progression complicates the task of identifying errors for AI trainers, hence making the comparison tasks crucial to RLHF more complex. This complexity highlights a fundamental challenge of RLHF: as models exceed human capabilities in knowledge, the gap in providing effective feedback widens.
To mitigate this challenge, OpenAI has trained CriticGPT to articulate critiques, shedding light on inaccuracies in ChatGPT’s responses.
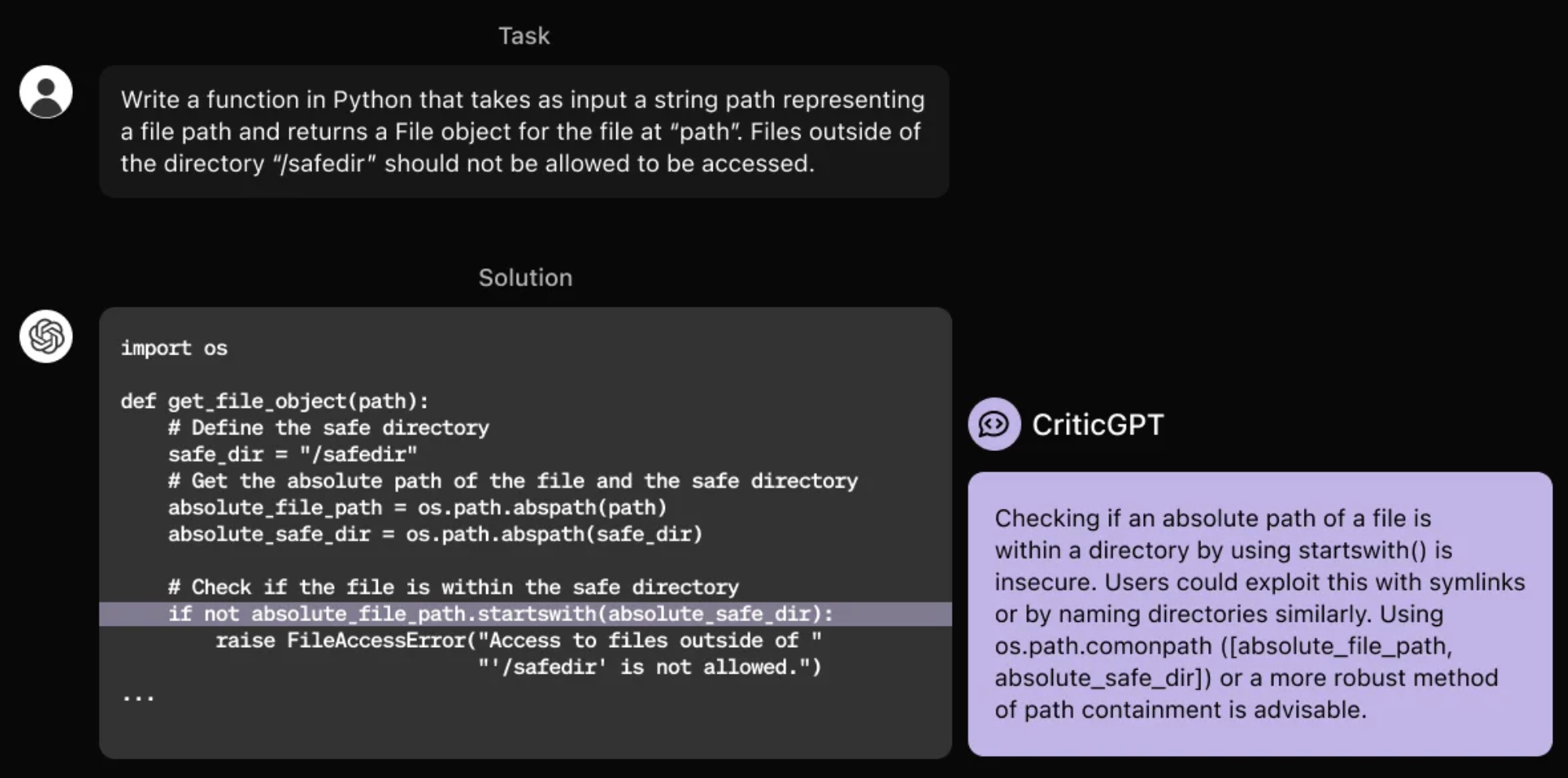
While not always flawless, CriticGPT significantly enhances the ability of trainers to detect a broader array of issues in model-generated content beyond what would be possible without AI support.
Additionally, the collaborative use of CriticGPT empowers users, enabling them to conduct more comprehensive critiques than they could independently. Furthermore, it reduces the incidence of ‘hallucinated bugs’ as opposed to scenarios where the model is used in isolation. In testing, critiques generated by the Human+CriticGPT team were preferred over those from solo trainers in over 60% of cases.
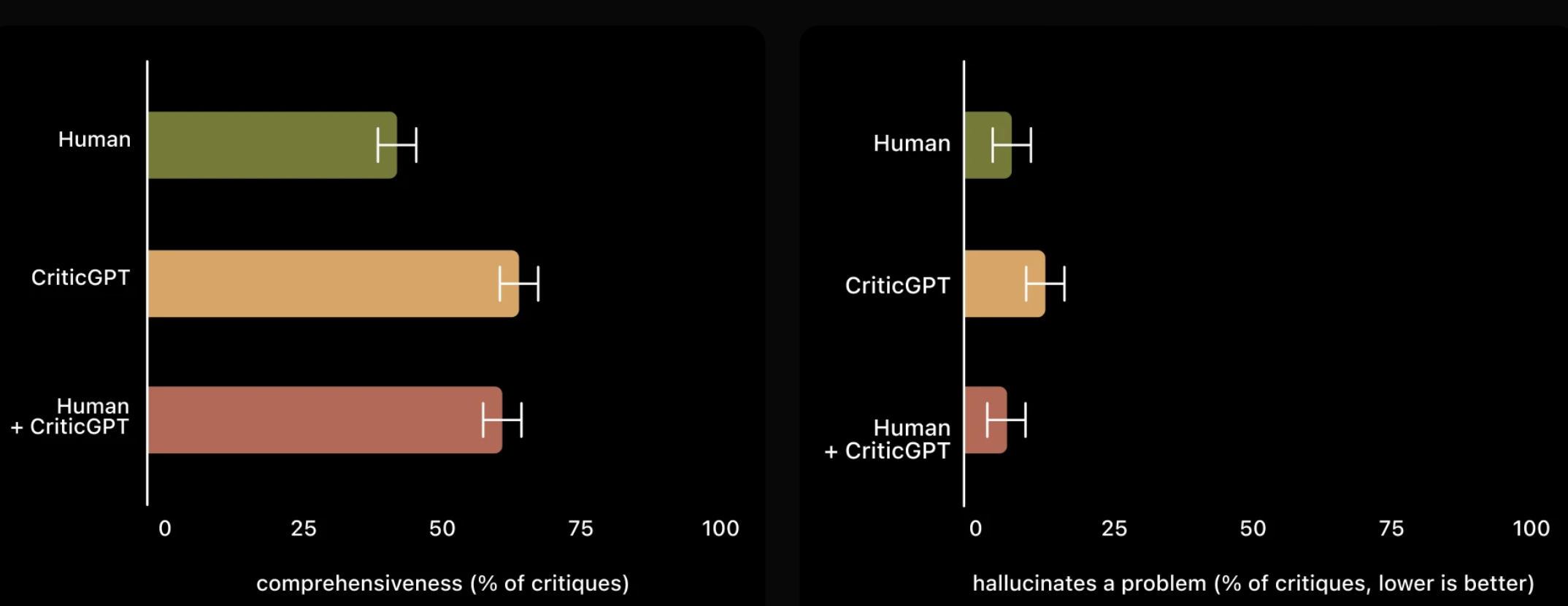
Methodology
The training of CriticGPT, akin to that of ChatGPT, took place through Reinforcement Learning from Human Feedback (RLHF), with the notable difference being its exposure to inputs robustly peppered with deliberate errors for critique. Trainers manually inserted these flaws into ChatGPT-generated code and fabricated feedback as though they had uncovered these mistakes themselves. Subsequent critiques of the adulterated code by the same trainer facilitated easy identification of accurate bug detection. Evaluation of CriticGPT’s efficiency took into account its adeptness at identifying both artificially introduced and pre-existing errors in ChatGPT outputs. In instances involving inherent faults, trainers showed a preference for CriticGPT’s analyses over ChatGPT’s in 63% of cases, attributed to a reduction in trivial criticisms and fewer hallucinated issues.
The leverage of additional test-time search against the critique reward model enabled the generation of longer and more cogent critiques. This approach allowed for a tailored balance between stringency in error detection and the precision-recall trade-off concerning hallucinations versus detected errors, culminating in critiques that significantly bolster RLHF effectiveness. The research document provides a comprehensive overview of these findings.
Challenges
CriticGPT’s training primarily focused on shorter ChatGPT responses. To supervise upcoming agents effectively, strategies are needed to aid trainers in navigating long and intricate tasks. The tendencies for models to generate erroneous outputs and for trainers to occasionally endorse these inaccuracies due to such hallucinations persist. Real-world errors frequently span across several segments of a response, whereas the current study centered on pinpointing mistakes locatable within a singular context. Future endeavors must tackle these widespread inaccuracies. Even with CriticGPT’s support, the sheer complexity of certain tasks or responses may overwhelm even the most seasoned experts.
Future Directions
Advancing the alignment of increasingly sophisticated AI systems calls for enhanced tools. Investigations into CriticGPT indicate promising prospects for applying RLHF to GPT-4, aiming to uplift human capability in generating more precise RLHF data for GPT-4. Efforts to extend and materialize this research are ongoing.
Discover more:
OptiPrime – Global leading total performance marketing “mate” to drive businesses growth effectively. Elevate your business with our tailored digital marketing services. We blend innovative strategies and cutting-edge technology to target your audience effectively and drive impactful results. Our data-driven approach optimizes campaigns for maximum ROI.
Spanning across continents, OptiPrime’s footprint extends from the historic streets of Quebec, Canada to the dynamic heartbeat of Melbourne, Australia; from the innovative spirit of Aarhus, Denmark to the pulsating energy of Ho Chi Minh City, Vietnam. Whether boosting brand awareness or increasing sales, we’re here to guide your digital success. Begin your journey to new heights with us!